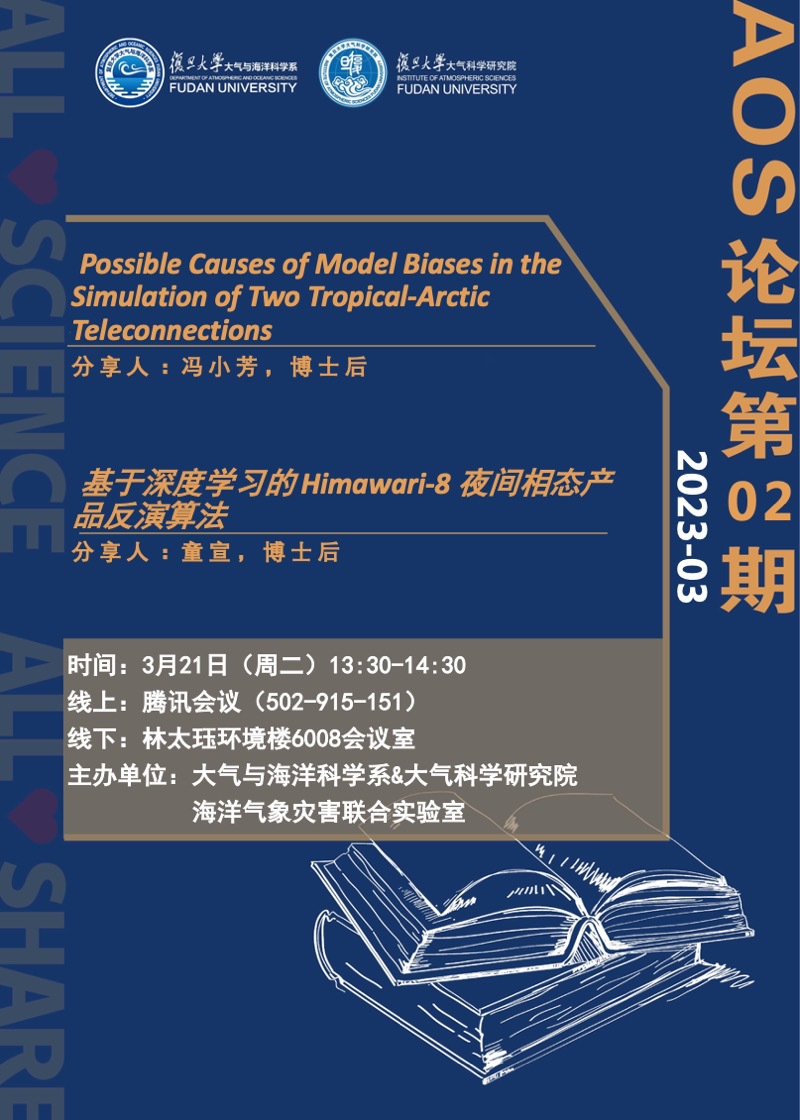
题目:Possible Causes of Model Biases in the Simulation of Two Tropical-Arctic Teleconnections
报告摘要:
The central role of tropical sea surface temperature (SST) variability in modulating Northern Hemisphere (NH) extratropical climate has long been known. However, the prevailing pathways of teleconnections in observations and the ability of climate models to replicate these observed linkages remain elusive. Here, we reveal two co-existing tropical-extratropical teleconnections albeit with distinctive spatiotemporal characteristics. The first mode, resembling the Pacific-North American (PNA) pattern, favors a Tropical-Arctic in-phase teleconnection (warm-Pacific-warm-Arctic) in boreal spring and winter. However, the second mode, with a seasonal preference of summer, is manifested as an elongated Rossby-wave train emanating from the tropical eastern Pacific that features an out-of-phase relationship (cold-Pacific-warm-Arctic) between tropical Pacific SSTs and temperature variability over the Arctic (referred to as the PARC mode). While climate models participating in CMIP6 appear to successfully simulate the PNA mode, the majority of models’ skill in reproducing the PARC mode is obstructed to some extent by biases in simulating low-frequency SST and rainfall variability over the tropical eastern Pacific and the climatological mean flow over the North Pacific during boreal summer. Considering the contribution of the PARC mode in shaping low frequency climate variations from the tropics to the Arctic, improving models’ capability to capture the PARC mode is essential to reduce uncertainties associated with decadal prediction and climate change projection over the NH.
个人简介:冯小芳,复旦大学大气与海洋科学系博士后。2021年博士毕业于南京信息工程大学,2018-2021年美国加州大学圣巴巴拉大学联合培养博士研究生。研究兴趣:台风气候、热带-中高纬相互作用、古气候等。
题目:基于深度学习的 Himawari-8 夜间相态产品反演算法
报告摘要:
云相态反演在卫星遥感和下游应用中具有至关重要的作用。然而,目前缺乏高效的夜间相态数据产品。本研究提出了一种融合残差神经网络和 Unet 神经网络的 Res-Unet34 算法,用于基于热红外波段反演 Himawari-8 的夜间相态产品。与 CALIPSO 和 MODIS 数据对比发现,通过 Res-Unet34 算法反演得到的云相态数据精度与Himawari-8 官方提供的日间云相态数据精度相当。此外,与广泛使用的随机森林相比,ResNet-34 反演速度更快且更准确。
个人简介:童宣,复旦大学博士后,2021年博士毕业于中国科学院大气物理研究所,随后就职于上海期智研究院工作一年。2022年10月进入复旦大学大气科学系从事博士后研究工作。研究方向为利用机器学习和深度学习进行卫星数据反演以及华北夏季降水诊断和预报。